Cloud observability is fast becoming an imperative as more organizations adopt multicloud IT strategies. To adapt, many are turning to AIOps and other automation technologies to solve the complex issues that accompany cloud-native architecture. Automation and AIOps for cloud-native environments can help IT pros prioritize issues and reduce false-positive alerts.
Still, as many organizations have discovered, AI and automation are broad terms, and solutions vary widely.
As part of Dynatrace’s 2022 Perform event, Dynatrace’s Illana Labuschagne, portfolio manager of ACE services joined David Jones, director of sales engineering, and Joel Alcon, director of product marketing to discuss how different approaches to AI for multicloud monitoring can impact operations.
Multicloud complexity obscures cloud observability
While shifting to a multicloud model offers many advantages, the change also introduces more data and systems to track and a complex matrix of systems to manage. “Observability has become a key capability for tackling this cloud complexity,” Alcon said.
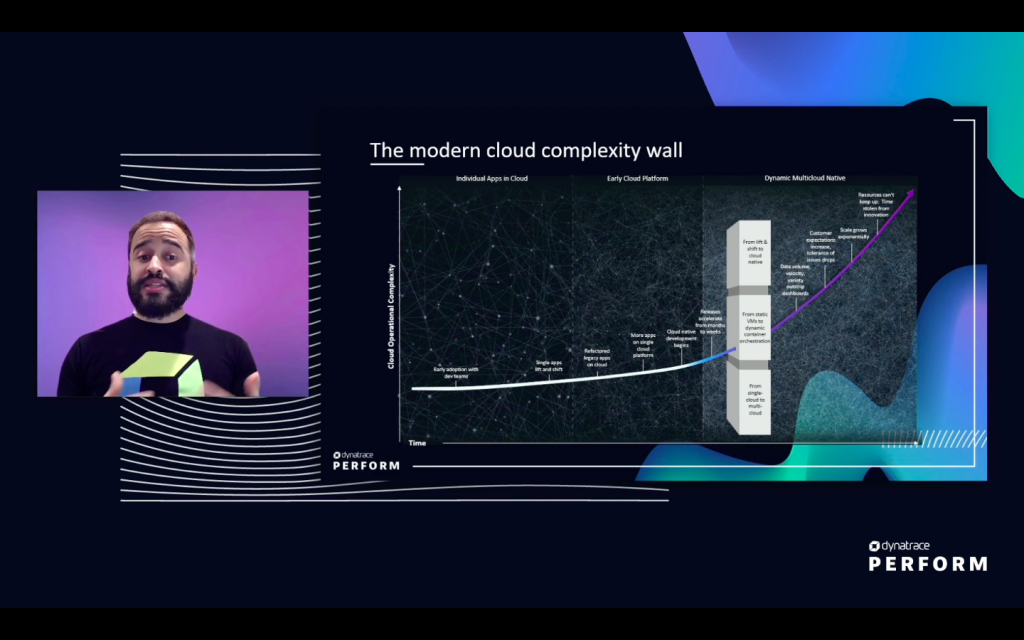
However, organizations often use a traditional approach to observability that relies on statistical correlation to detect performance or security problems. Just correlating statistics leaves analysts with a lot of manual work to verify precisely where and why a problem has occurred. This traditional approach to observability lacks the specificity needed to keep pace with cloud-native environments and automate DevOps processes.
Jones identified two types of complexity:
- Accidental complexity. The result of adding new technology without planning for the overhead, such as introducing new dashboards or messaging services, or adopting multiple cloud vendors without the infrastructure to manage it.
- Planned complexity. A measured approach to adding new technology to your stack, such as containerizing applications.
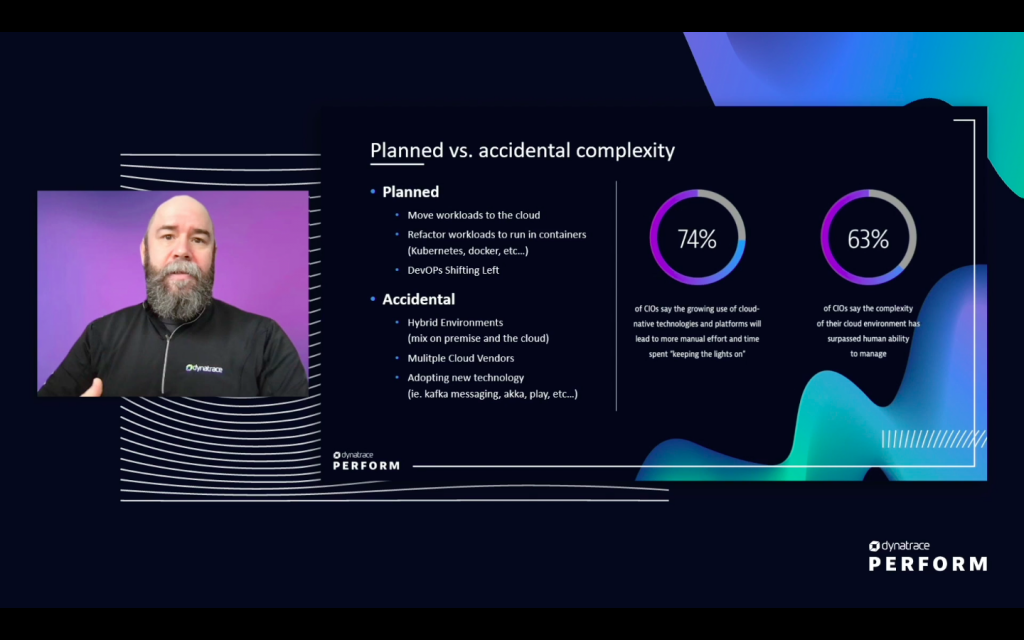
According to studies from Dynatrace, 74% of CIOs believe moving to cloud-native technologies will increase manual overhead at their organizations. 63% say their cloud environments have already surpassed a human’s ability to manage.
Two approaches to AIOps
“In the AIOps world, there are a couple different approaches to AIOps,” Jones explains: machine-learning AIOps and deterministic, or causal AIOps.
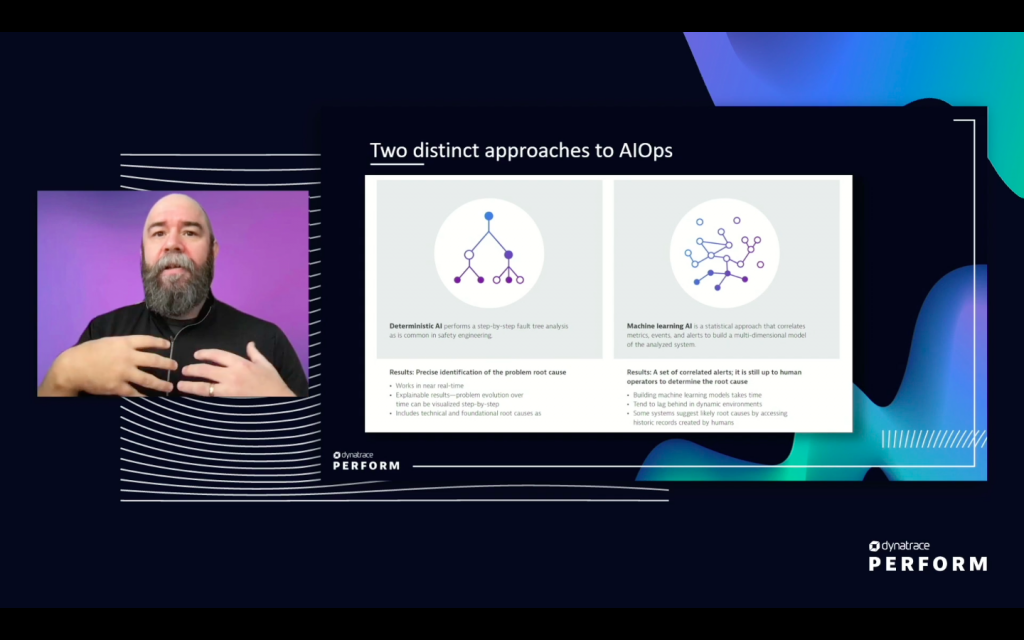
Machine learning-driven AIOps
The machine-learning approach is designed to find patterns and correlations in data. But when it comes to multicloud systems, correlation does not always align with causation. Causation is even more difficult to identify as ephemeral workloads increase. Workloads come in and out of existence quickly, which means the landscape for finding patterns is constantly changing.
Deterministic AIOps
An approach that uses deterministic or causal AI works off a dynamic model of how a system operates, where AI is not attempting to find a pattern. Rather, the pattern is the existing stack, and deterministic AI is already aware of this pattern in real time.
A deterministic AIOps approach uses this dynamic architectural pattern as the foundation of a “smart” solution. This approach allows the AI to determine the precise root cause of issues based on the observed behavior of components in the stack. With this type of model, AIOps has context embedded as a foundational part of the solution itself.
AIOps brings clarity of intelligence and automation to cloud observability
Lastly, Labuschagne discussed the ultimate goals of AIOps to cloud observability: clarity of software intelligence and automation. Properly contextualized information gives teams the ability to automate various pipelines, such as a DevOps continuous validation process.
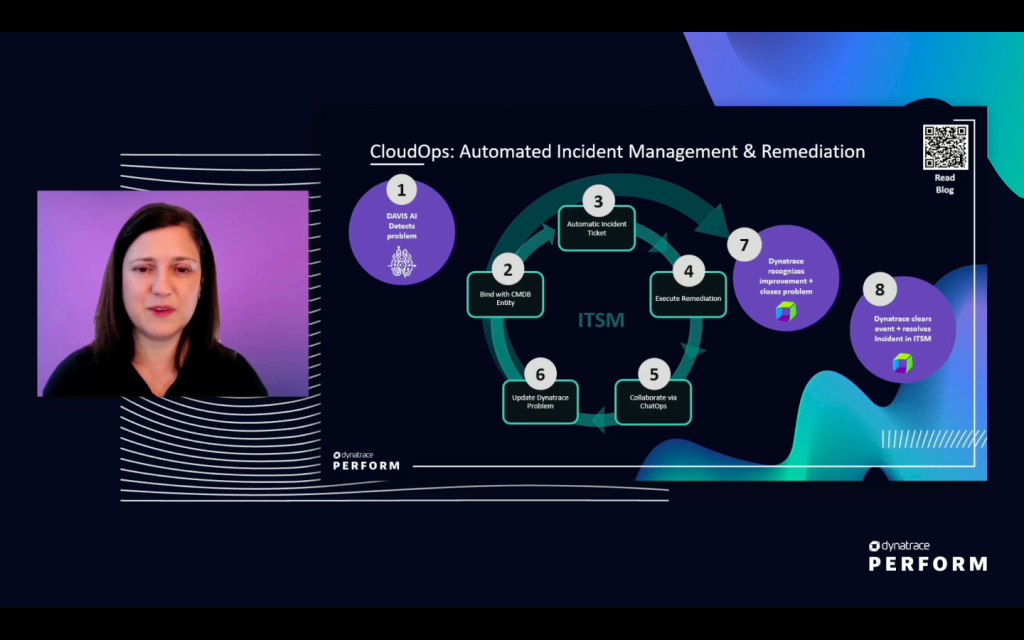
Labuschagne highlighted the ability to implement automated quality gates given the deterministic model’s ability to contextualize a multicloud component function. In the end, she stressed that this technology is about increasing overall operational efficiency, from the development lifecycle to SecOps (or the combined efforts of security and operations teams).
For more about AIOps and its impact on multicloud platforms, check out the full session, How Dynatrace AIOps powers cloud observability to optimize your apps across multi-cloud platforms. You can find more sessions from the Perform event are also available at the on-demand watch site.
For more coverage of Dynatrace Perform 2022, check out our Dynatrace Perform 2022 conference coverage guide Themes to watch at Dynatrace’s annual conference.
Looking for answers?
Start a new discussion or ask for help in our Q&A forum.
Go to forum